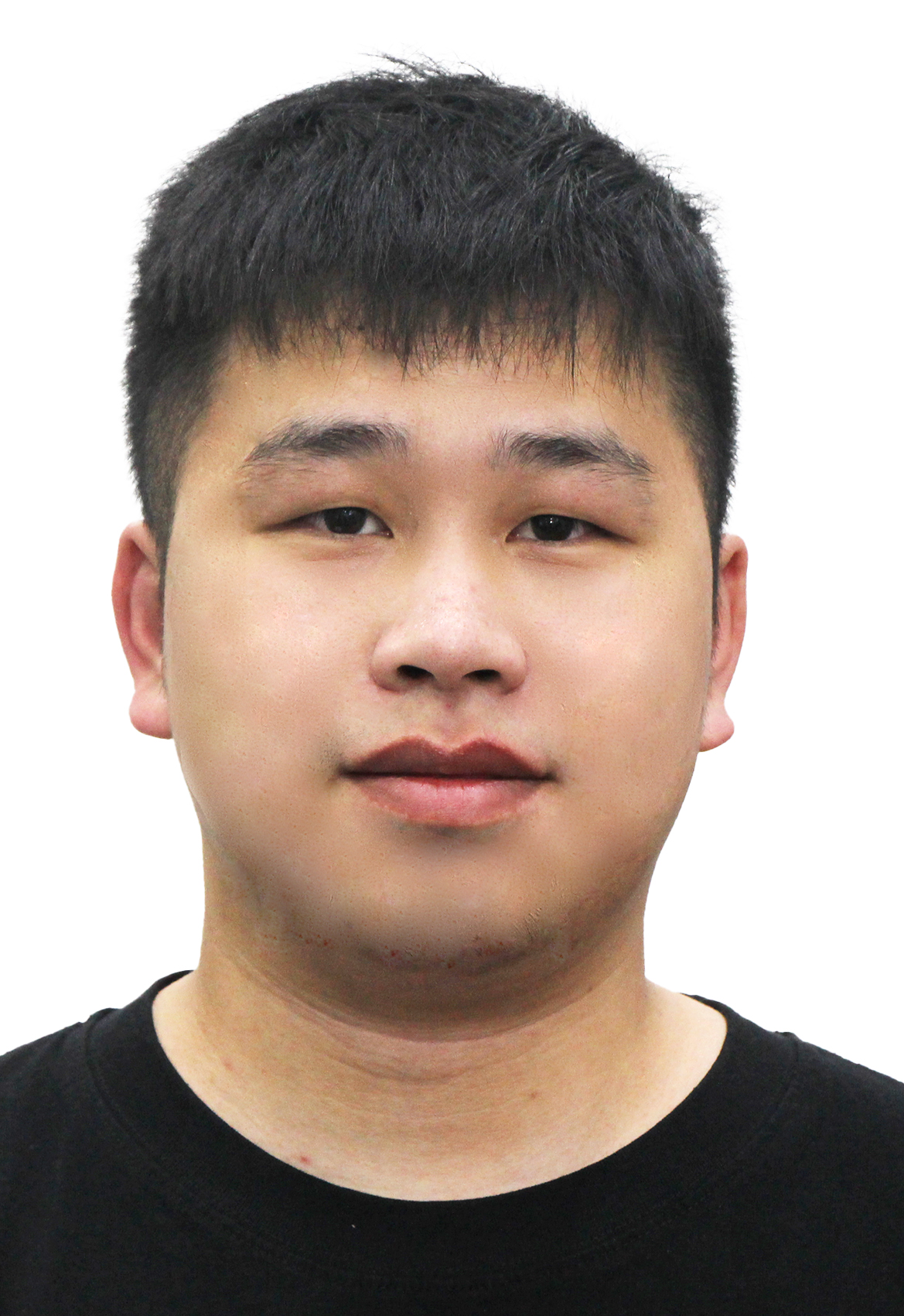
CV / Google Scholar / GitHub
Email: zhanpeng at cs.columbia.edu
I am a Ph.D candidate in computer science at Columbia University. My research focuses on robotics, computer vision and reinforcement learning. I am privileged to be co-advised by Professor Matei Ciocarlie and Professor Shuran Song. I am also a member of the Robotic Manipulation and Mobility (ROAM) Lab and the Columbia Artificial Intelligence and Robotics (CAIR) Lab.
Before joining CU, I recieved a master's degree from University of Southern California, where I worked as a research assistant at the Robotic Embedded System Laboratory (RESL) and advised by Professor Gaurav Sukhatme. When I am not working, I enjoy cooking, brewing coffee and playing with my dog Nova. Here is a picture of Nova being corrupted by Gaussian noises.
Research
I'm a full-stack roboticist -- I believe robotics must be solved through progress in many different integral components. While scaling up data collection is important, we also need to design the right hardware (e.g, manipulators and contact sensors) and learning algorithms (e.g., learning from imbalanced sensor data) to enable robust policies on real robots for contact-rich tasks. To this end, my research includes:
- Policy learning (e.g., imitation learning, reinforcement learning) for contact-rich tasks
- Hardware design (e.g., manipulators, data collection systems, and contact sensors) and task-driven hardware optimization
- Learning from multi-sensor and imbalanced data
- Human-in-the-loop policy learning and deployment
My full research statement will be available soon.
News
- [Jun 2025] We gave several demos of our newly designed ROAMHand3, equipped with our dynamic tactile sensors and F/T sensors in several places, including: NEMS2025, Columbia AI Summit 2025, and General Motor Innovation Day 2025.
- [May 2025] Our paper on active acoustic tactile sensing is accepted by IROS 2025.
- [Apr 2025] I gave an invited talk at the CAIT 2025 Spring Symposium. Title: Robotic Manipulation using Low-Cost Force/Torque Sensing.
- [Sep 2024] I gave an invited talk at the MIT CSL Seminar. Host: Pulkit Agrawal.
- [Jul 2024] I gave two invited talks with the title "Co-designing Robots with Reinforcement Learning" at RSS 2024 workshop on Embodiment-aware Robot Learning and ICRA 2024 co-design workshop.
- [Jun 2024] Our paper about optimizing underactuated tentacle robots is accepted by IROS 2024.
- [Jan 2024] Two papers (hardware-policy co-design and learning human-in-the-loop policy) are accepted by ICRA 2024.
- [Jan 2023] My intership project Pick2Place is accepted by ICRA 2023!
- [Jun 2022] I started my summer internship as a research intern at Samsung AI Center NY.
- [May 2022] DiscoSyn and UMPNet are presented at ICRA 2022!
Publications (* - indicates equal contributions and co-first authors)
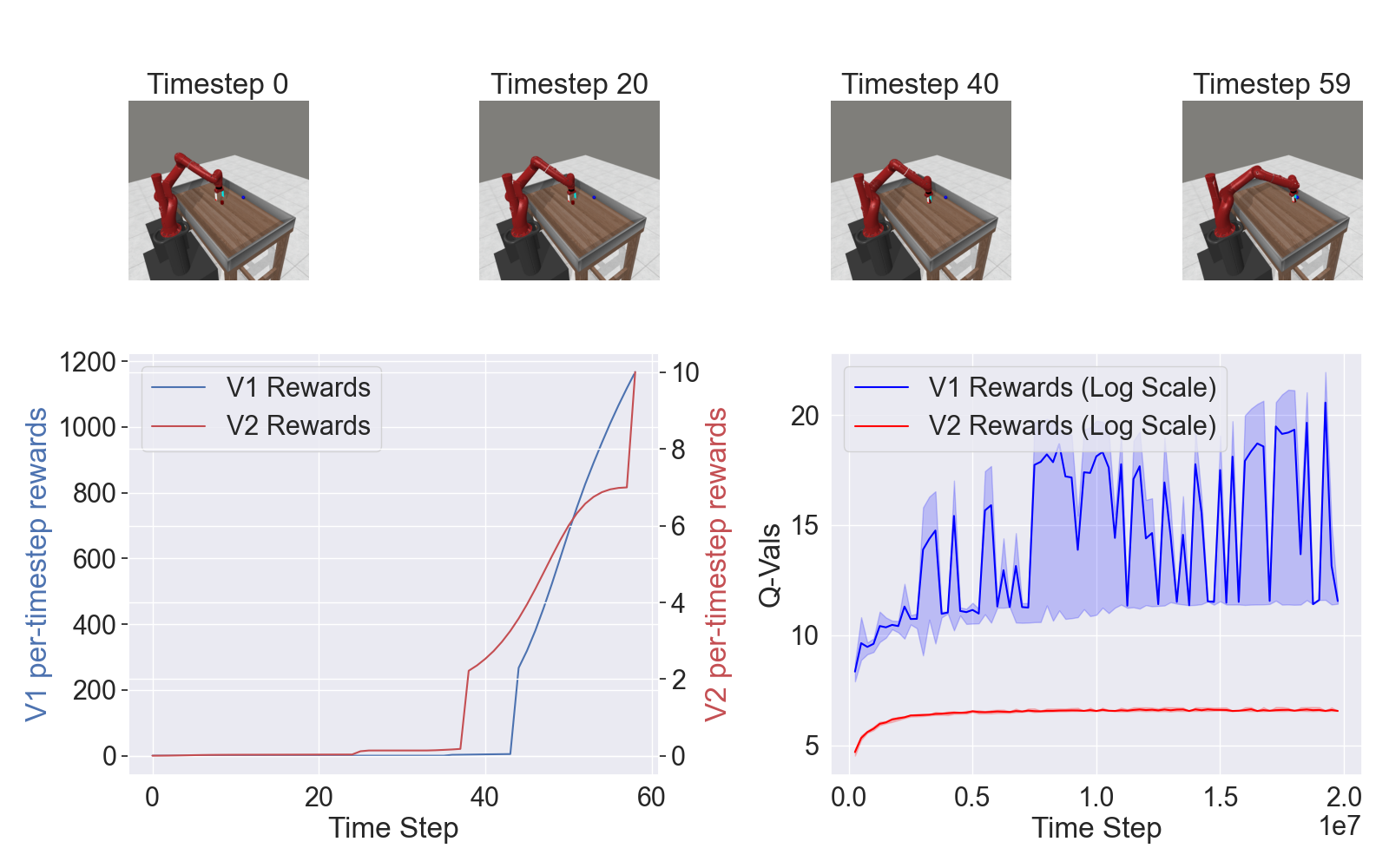
Under review. This is an updated version of our previous work Meta-World.
Paper
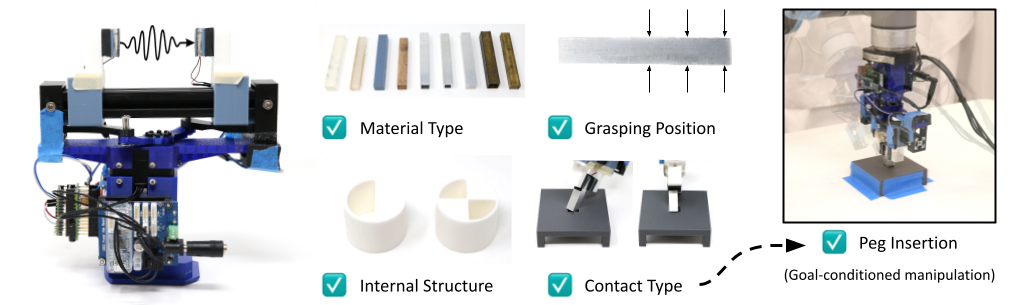
IEEE/RSJ International Conference on Intelligent Robots and Systems (IROS) 2025
Paper
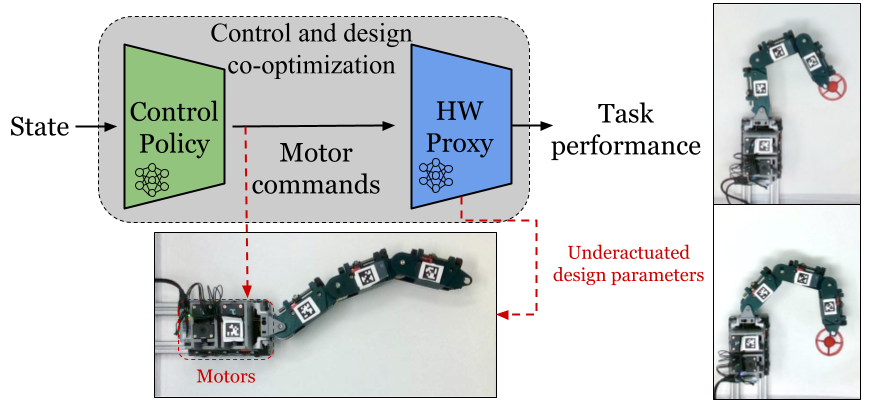
IEEE/RSJ International Conference on Intelligent Robots and Systems (IROS) 2024
Webpage /
Paper
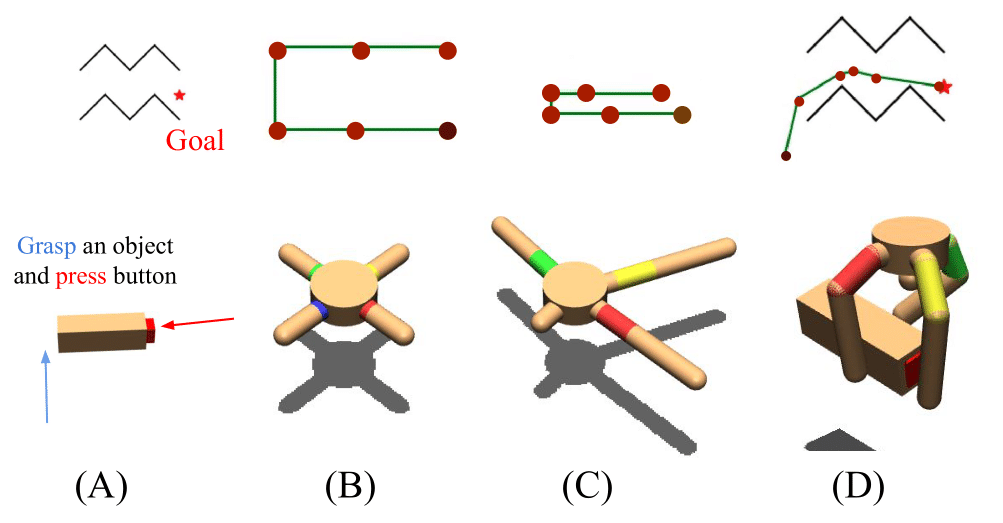
International Conference on Robotics and Automation (ICRA) 2024
Webpage /
Paper
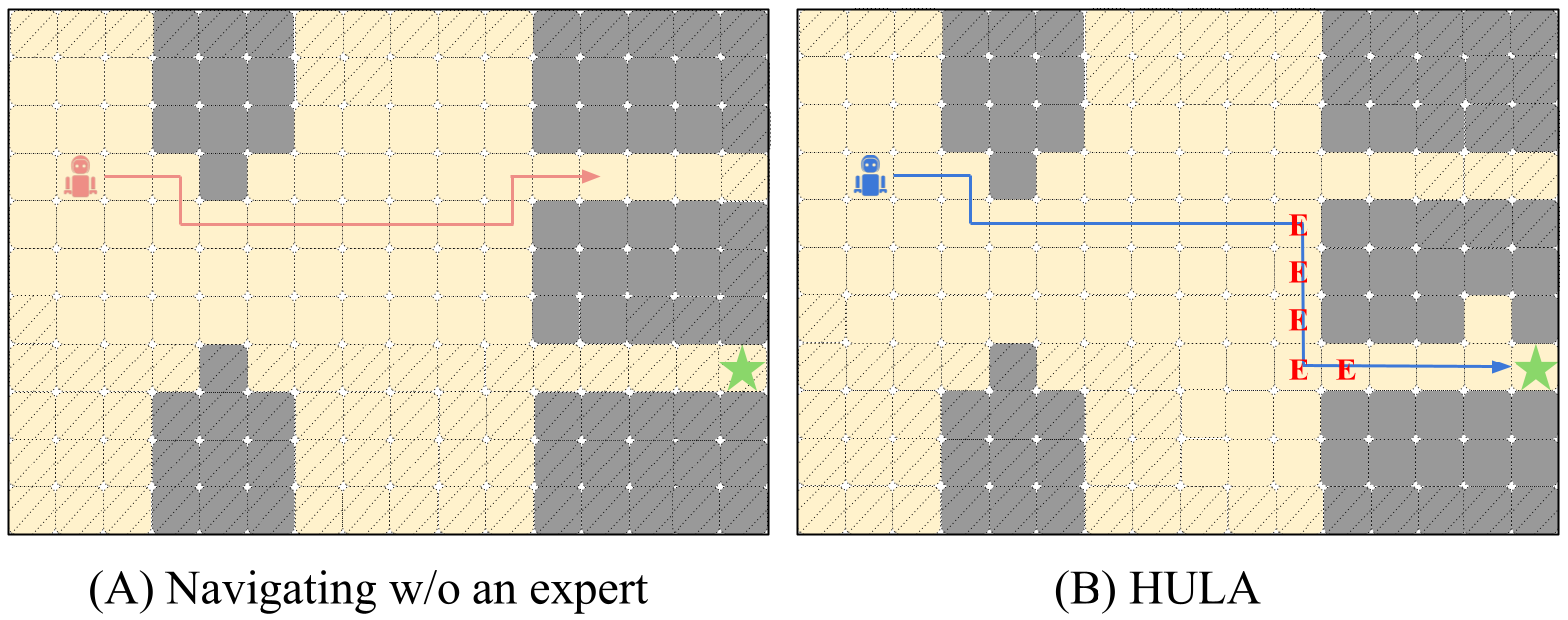
International Conference on Robotics and Automation (ICRA) 2024
Webpage /
Paper
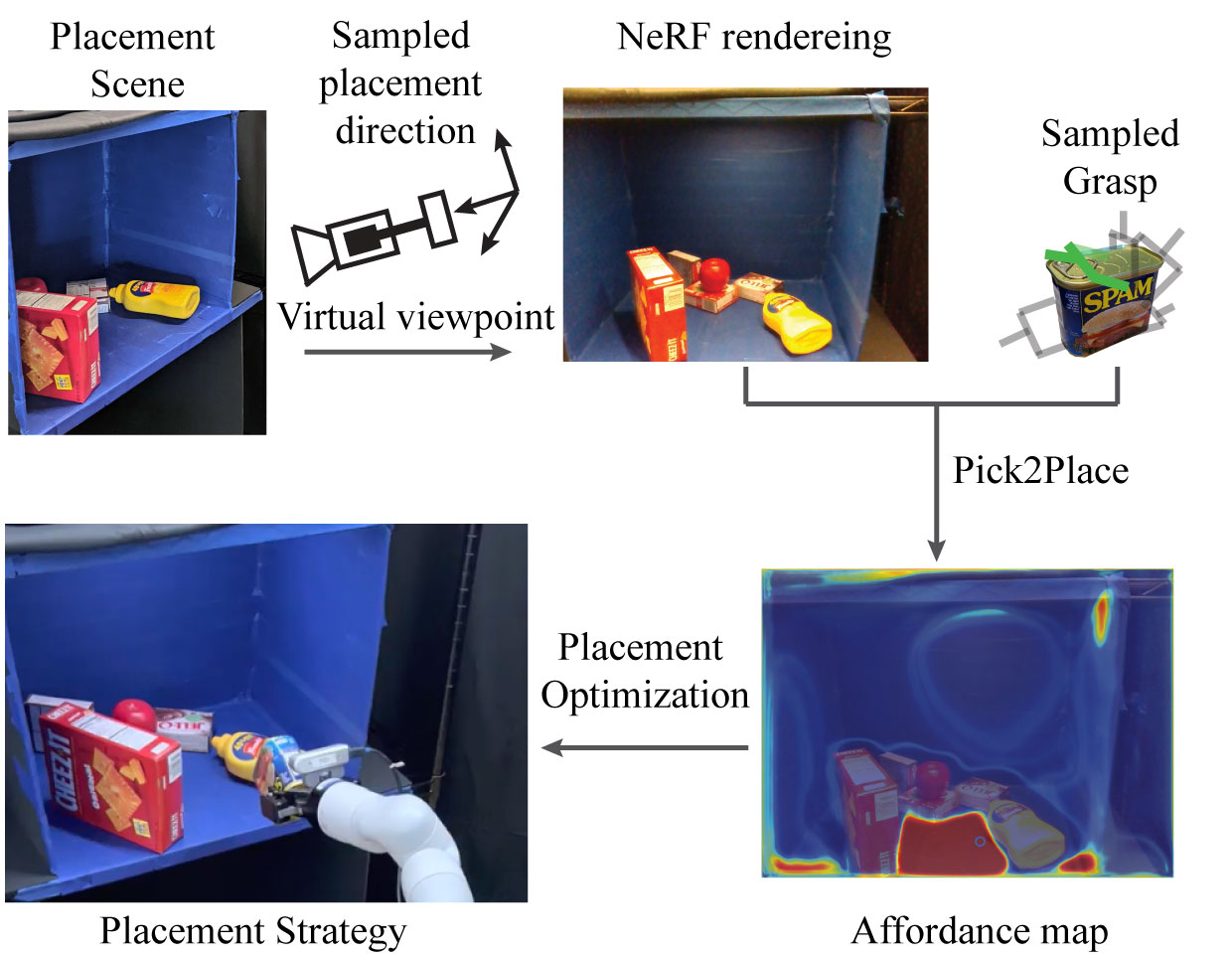
International Conference on Robotics and Automation (ICRA) 2023
Paper
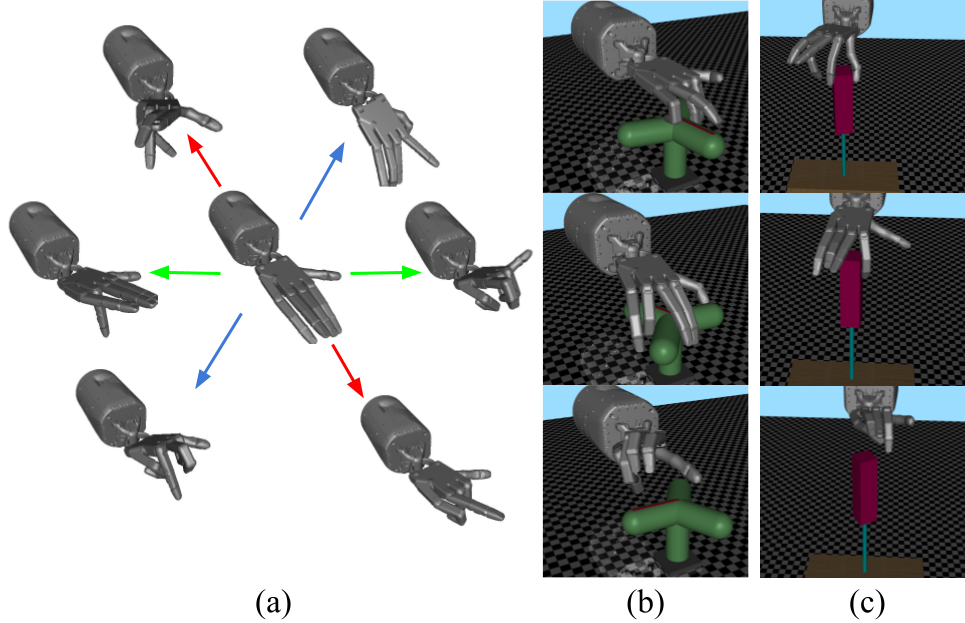
International Conference on Robotics and Automation (ICRA) 2022
Webpage /
Paper /
Codes
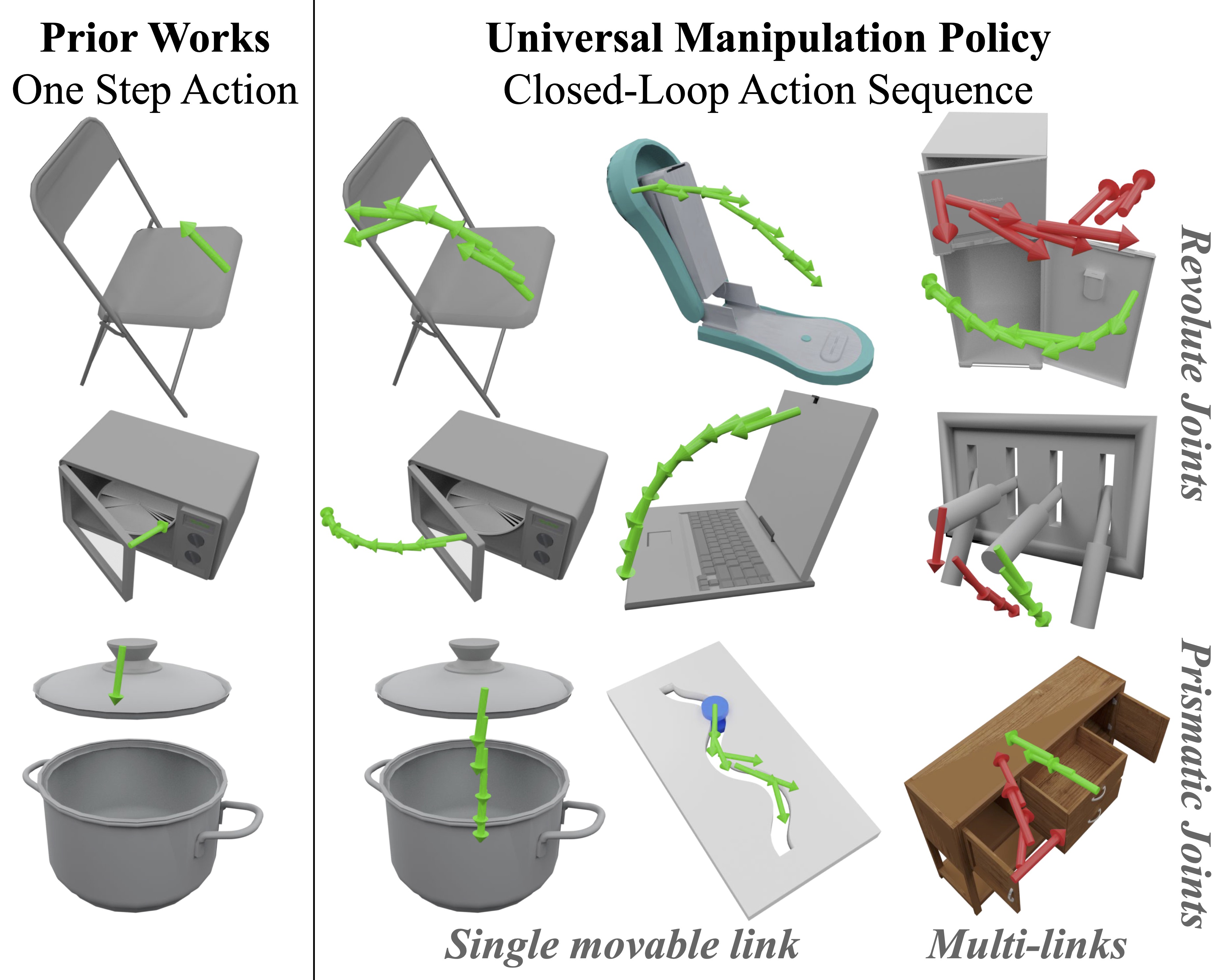
Robotics and Automation Letters (RA-L)
International Conference on Robotics and Automation (ICRA) 2022
Webpage /
Paper /
Codes
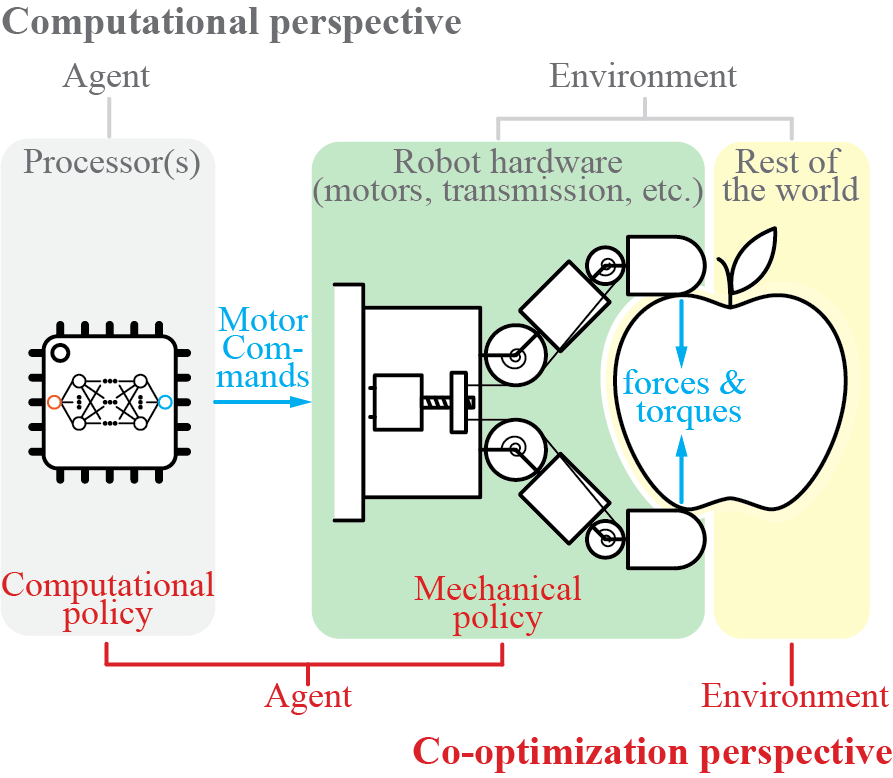
*,
*,
and
Science Robotics
Conference on Robot Learning (CoRL) 2020
Webpage /
Paper /
Science Robotics Article
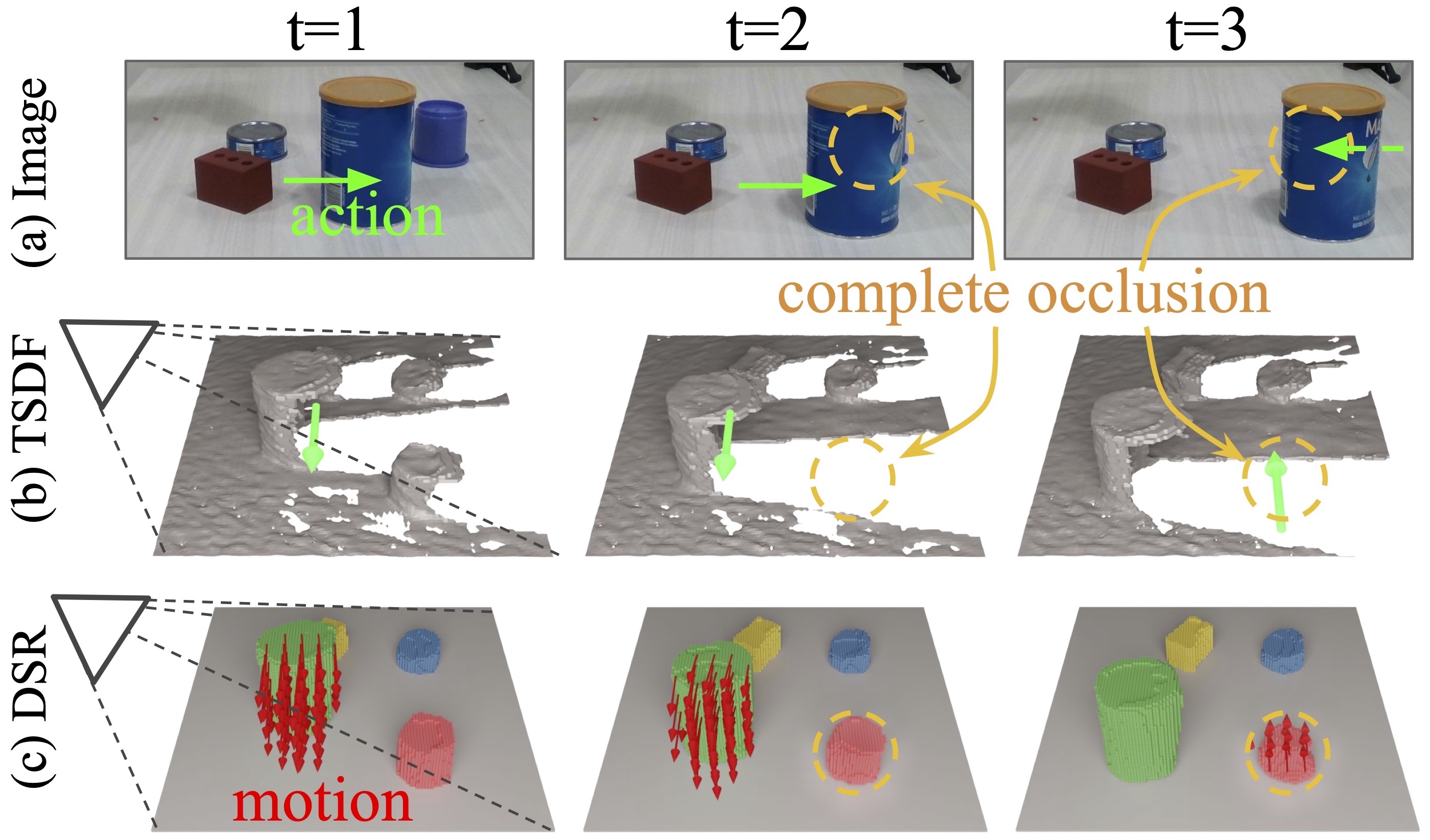
*,
*,
,
and
Conference on Robot Learning (CoRL) 2020
Webpage /
Paper /
Codes
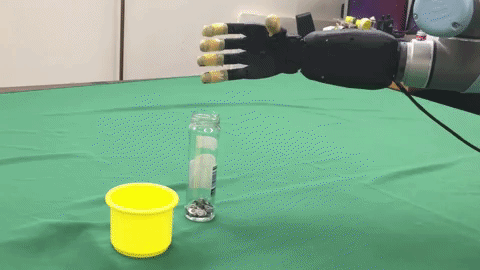
,
and
International Conference on Intelligent Robots and Systems (IROS) 2020
Webpage /
Paper /
Video
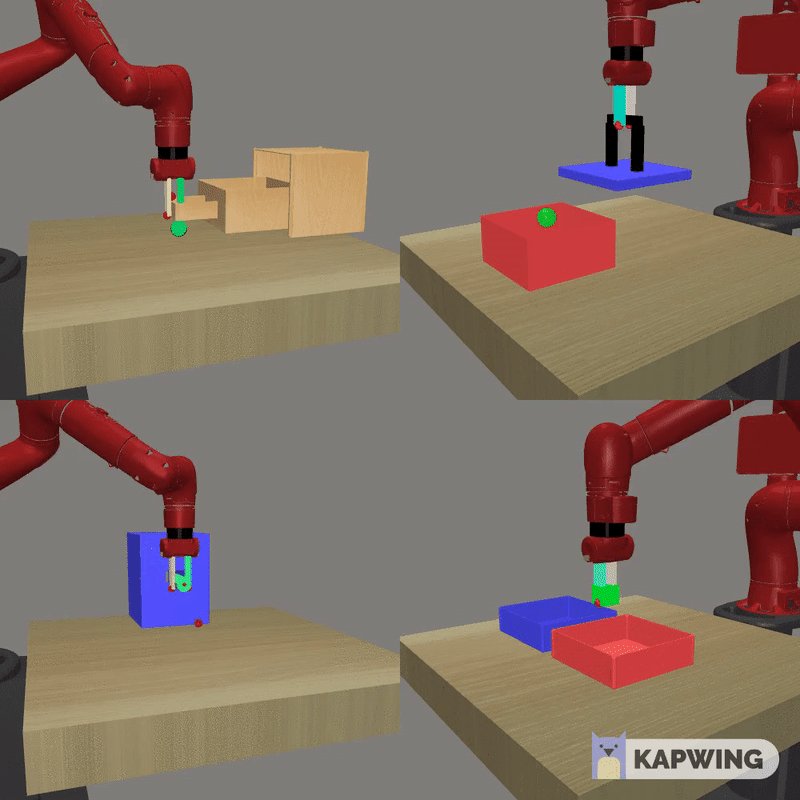
*,
*,
*,
,
,
,
and
Conference on Robot Learning (CoRL) 2019
Webpage /
Paper /
Codes
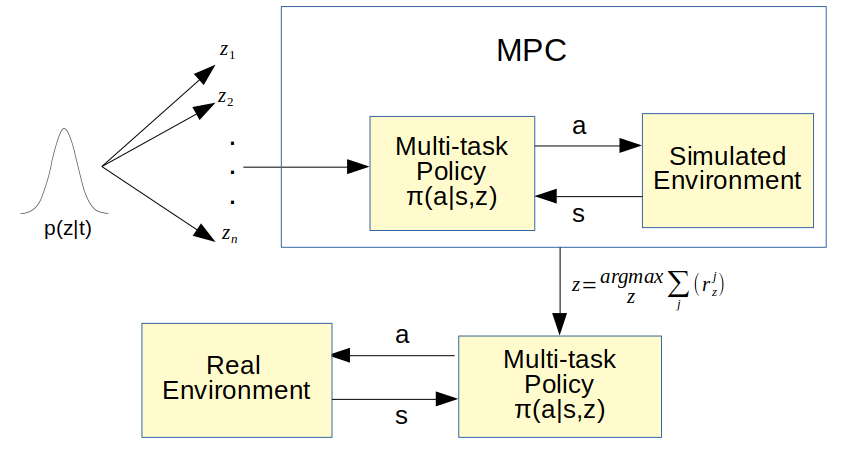
*,
,
,
,
,
,
and
Neural Information Processing Systems (NIPS) 2018
Paper /
Codes
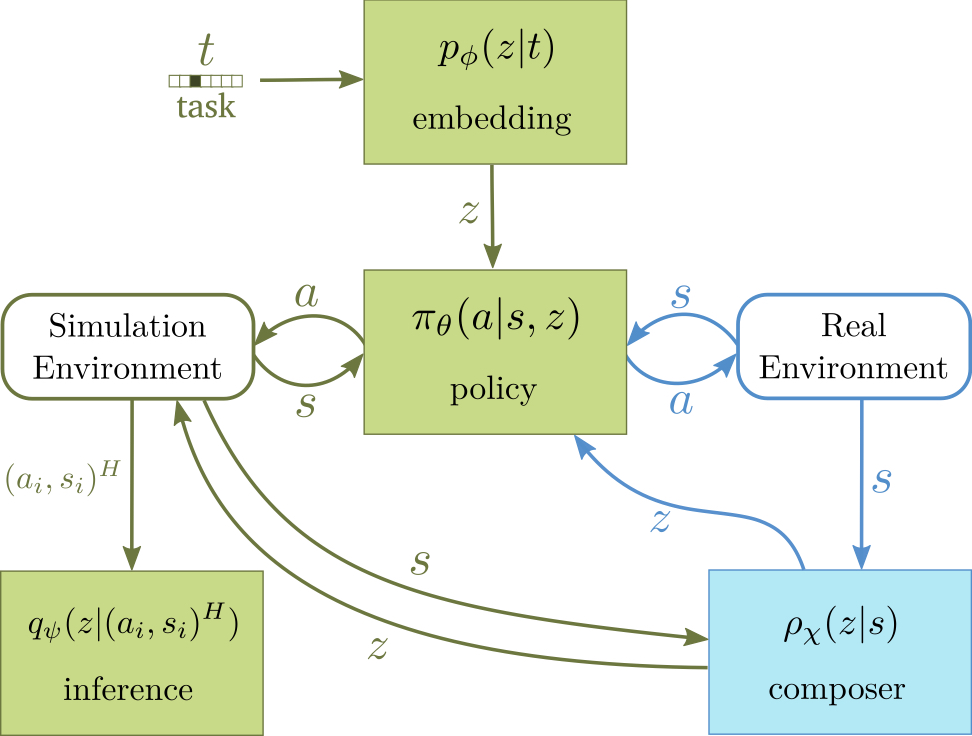
*,
*,
,
,
,
,
,
and
The International Journal of Robotics Research (IJRR)
International Symposium on Experimental Robotics (ISER) 2018
Paper /
Codes /
Video /
Journal version
Mentoring, Service, and Teaching
Currently, at Columbia, I have the luck to work with: Hua-Hsuan Liang (MS in CS), Edward Zhang (UG in CS), and William Wang (UG in CS). Previously, I mentored:
- Yifeng Cao (now Ph.D at Virginia Tech)
- Andrew Liu (now AI intern at Flagship Pioneering)
- Toby Kreiman (now Ph.D at UC Berkeley)
- Siddharth Singi (now ML Scientist at Memorial Sloan Kettering Cancer Center)
- Alvin Pan (now AI Scientist at Faction Imaging Inc.)
- Rohan James (now MLE at AWS)
I have been a long-term (>= 4 years) reviewer for several conferences and journals, including: CoRL, ICRA, IROS, RSS, RA-L, TMECH, IJRR, and T-RO. Other than that, I have several teaching experiences:
- Invited Lecturer: MECE 6616 Robot Learning 2024Spring, 2025Spring
- Teaching Assistant: MECE 6616 E Robot Learning 2020Spring, 2022Spring
- Teaching Assistant: CS111 Introduction to Computer Science
Softwares
I was a member of rlworkgroup and took part in development of several robot-learning-related open-source projects.
- Garage: A toolkit for reproducible reinforcement learning research.
- Dowel: A little logger for machine learning research.
- Meta-World: A collection of environments for benchmarking meta-learning and multi-task reinforcement learning algorithms.